Leah Pistorius
March 1, 2022
Human-Centered Data Science: An Introduction, a new book authored by Cecilia Aragon, Professor in the Department of Human Centered Design & Engineering, is out now through MIT Press.
In the book, Aragon and co-authors Shion Guha, Marina Kogan, Michael Muller, and Gina Neff, explore best practices for addressing the bias and inequality that may result from the automated collection, analysis, and distribution of large datasets.
In a panel discussion hosted by the Minderoo Centre for Technology and Democracy, the co-authors describe the new interdisciplinary field of human-centered data science. They offer a brief and accessible overview of many common statistical and algorithmic data science techniques, explain human-centered approaches to data science problems, and present practical guidelines and real-world case studies to help readers apply these methods. View the panel recording below.
Recorded February 25, 2022 | Panel transcript available here
View the book
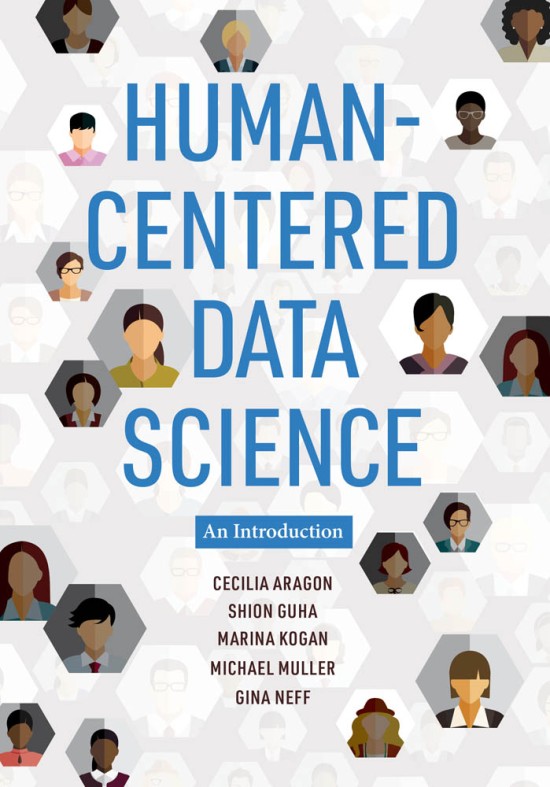
Human-Centered Data Science: An Introduction
By Cecilia Aragon, Shion Guha, Marina Kogan, Michael Muller and Gina Neff
About the book
Human-centered data science is a new interdisciplinary field that draws from human-computer interaction, social science, statistics, and computational techniques. This book, written by founders of the field, introduces best practices for addressing the bias and inequality that may result from the automated collection, analysis, and distribution of very large datasets. It offers a brief and accessible overview of many common statistical and algorithmic data science techniques, explains human-centered approaches to data science problems, and presents practical guidelines and real-world case studies to help readers apply these methods.
The authors explain how data scientists' choices are involved at every stage of the data science workflow—and show how a human-centered approach can enhance each one, by making the process more transparent, asking questions, and considering the social context of the data. They describe how tools from social science might be incorporated into data science practices, discuss different types of collaboration, and consider data storytelling through visualization. The book shows that data science practitioners can build rigorous and ethical algorithms and design projects that use cutting-edge computational tools and address social concerns.